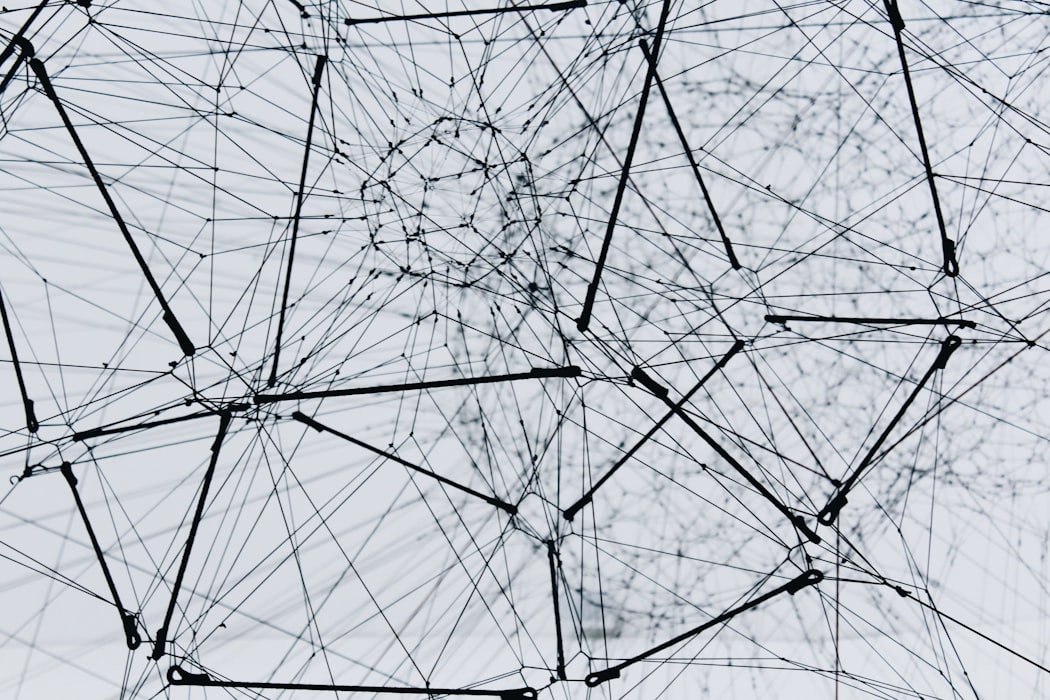
This is inspired by a paper “Topology matters: network topology affects outcomes from community ecology neutral models” by White and Kiester (2008). Here “(network) topology” basically means the arrangement or the structure of systematically sampled points. Or understand it like this: what is the relationship between neighboring points, and how would points be considered as neighbors.
This paper typically tested this in the context of ecology: whether the topology of a network of ecological communities affects the dispersal statistics of populations in the communities. To do this, the paper compared three tessellations, hexagon tessellation, square tessellations using von Neumann neighborhoods (which neglects the proximity of the diagonal neighbors), and square tessellations using Moore neighborhoods (which includes the proximity of the diagonal neighbors), by examining the results of neutral ecology community models.
Results showed that higher degrees of adjacency lead to larger and more evenly distributed populations as dispersal increases. Differences among topologies increased as the probability of dispersal increased. So that hexagon tessellations have different performance than and are often superior to, square tessellations because both types of square tessellations have compromised adjacency relations.
However, the impacts of topology on models of ecological processes have been little studied, and there is little awareness of the possibility of important effects. When I did the simultaneous autoregressive models in one of my Master’s projects, I did systematic samples at 2 km intervals, which was a square tessellation. The model accounted for spatial structures on this base, i.e., adjacent relationships based on a lattice structure. I did that because I honestly never thought about a hexagonal tessellation – I guess hexagonal sampling is so less frequently used that only a small proportion of people in the ecological community would consider it as a way to go. If taking this alternative into account, it would mean that some of the concepts in geostatistics need to be updated, like the first and second-order neighbors. The equations of geostatistics, such as Moran’s I and Getis-Ord G*, will not be changed, but the spatial relationships, spatial structure, and weighting matrix may need redefinition. So do spatial models, such as autoregressive models and auto-covariate regression, whichever addresses spatial autocorrelation and accounts for the spatial matrix, would have to update the model fitting programming behind to include an alternative option of hexagonal tessellation. I am not sure how much it would change the modeling results, perhaps it varies in different modeling scenarios, but at least the potential differences need to be explored, quantified, and compared.