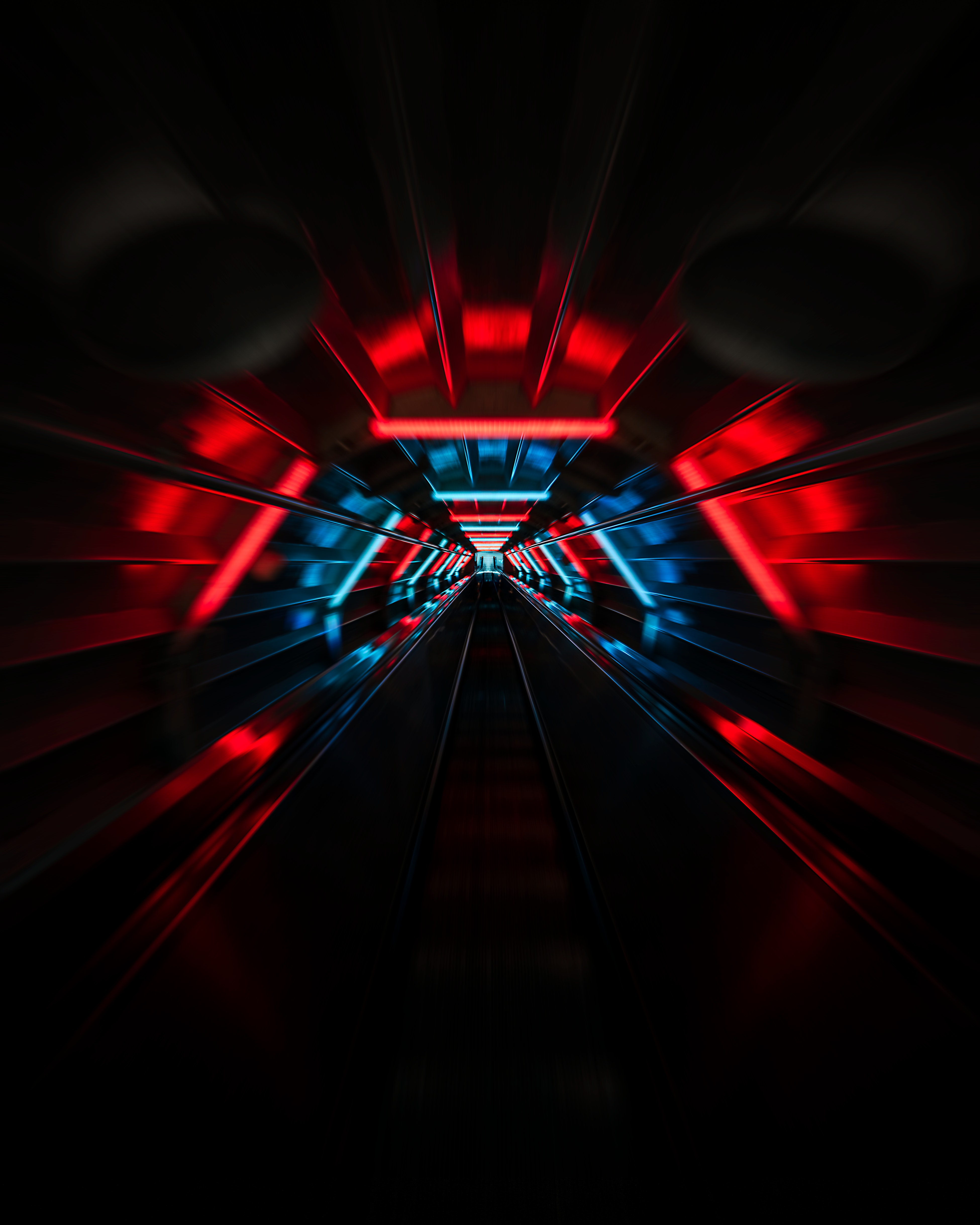
DGGS-powered datacubes
According to Purss et al. [1], DGGS and geospatial datacubes are indicating different aspects of the same stuff which is essentially congruent geography [2]. The term congruent geography means to represent every layer or theme by the same basic spatial units, and thus allows the geo-spatial data to be examined, analyzed, and visualized either horizontally or vertically [2]. As aforementioned in Section 1.3, a geospatial datacube can be built on a DGGS by adopting DGGS’s underlying spatial architecture. This is superior to a conventional datacube in terms of the spatial limitations or constraints when dealing with a global scale, and the representation or integration of the global spatial data [1]. Some projects have been working on the applications of DGGS-driven datacubes (e.g., [3, 4]), however, these projects are in progress and a lot of aspects of theories or applications still need further studying. For example, future research may focus on standardizing the implementation details, enhancing the ability to store multi-dimensional arrays, improving the quality of spatio-temporal data, addressing the spatial integrity constraints in spatial multi-dimensional databases, accelerating the accesses or queries, enabling advanced spatial-temporal analysis, developing appropriate service interfaces, and reaching large adoption and acceptance [1, 5-7].
Big data management through DGGS and cloud computing
In the era of big data in the Earth observation context, efficient management and computation of big data are of great need to turn the flood of raw data into understandable information. Recent research has proposed a solution to the global Earth observation data processing, which integrated cloud computing as the computing power and DGGS as the unified framework into a closed-loop [8]. Additionally, some countries or institutions have developed Open Data Cube to store, manage, and analyze Earth observation data, which made contributions to numerous environmental topics (e.g., [9]). Although it has been shown that integrating cloud computing techniques with DGGS provides opportunities to manage such big Earth observation data, there are potential challenges alongside [8]. First, grid indexing and data query methods need further improvement. Hierarchy-based, space-filling curve based and coordinate-based indexing mechanisms are currently most common for DGGS techniques [10]. These indexing approaches are well suited for spatial proximity emphasized circumstances but are not practical for distributed or parallel computation. Indexing methods compatible with distributed or parallel computation are in need [8]. Second, the extended DGGS framework with the time dimension needs further development. Earth observation data has been traditionally analyzed in two dimensions, while time-series analysis on Earth observation data has increasingly attracted researchers’ attention. Similar to space, analysis on the time dimension has the problems of partition and scale, which are more complicated to deal with in a variety of aspects [11]. Third, various models of processing Earth observation data have been developed previously, while the parallel implementations of these existing models are still challenging to realize. Furthermore, the integration with the cloud computing environment needs a more comprehensive study, considering the data analysis is based on the spherical reference system instead of a plane reference system [8].
Application of DGGS to sensor networks and the Internet of Things
The Internet of Things (IoT) was proposed to be an infrastructure that enables heterogeneously networked objects or devices to interact with each other [12]. As defined by the International Telecommunication Union (ITU) Standardisation Sector, IoT is “a global infrastructure for the information society, enabling advanced services by interconnecting (physical and virtual) things based on existing and evolving interoperable information and communication technologies” [13]. Relating to Digital Earth, a ‘Thing’ should have the characteristics of networked communication, programmability, and sensing or actuating capabilities [12]. According to Purss et al., DGGS have the potential to be applied as a common framework in the context of sensor networks, providing uniformed methods and protocols among different spatial data infrastructures [14]. Purss et al. [14] demonstrated the benefits of establishing international standards and enabling interoperability through the implementation of DGGS. Another key benefit of adopting DGGS for the Internet of Things (IoT) platforms is that DGGS tile the Earth into hierarchical sets of discrete cells instead of points, which can avoid the massive processes of interpolating sensor located points into understandable areal coverages [14]. Because an areal-phenomenon has more valuable information compared to the individual points, the usage of DGGS provides an opportunity to convert the vast volume of IoT data into meaningful information timely and dynamically. Research on the IoT with respect to the applications to Digital Earth is still in its infancy, so at least four aspects of research need to be investigated. First, future research on IoT should cover the discoverability, acquisition, and communication of geospatial information [12]. Second, the understanding of spatial objects and their relationships need to be improved, which includes the spatial analysis and the geospatial standards of “Things” [12]. Third, how IoT collaborating with Digital Earth benefits decision making in different application domains needs to be tested [12]. Further, research on developing DGGS-based IoT platforms is expected in the future [14].
Handling point clouds with DGGS
It has been known that integrating multi-source point clouds into a global point cloud suffers from several problems such as different reference systems and inconsistent levels of details of the point clouds. Recently, given its hierarchical, multi-resolution nature, the potentials of applying DGGS to managing massive global point clouds have been discussed [15]. In their study, DGGS were extended into 3D and 4D to explore the multi-dimensional point clouds, with the third dimension as the distance of a point to (above or below) the Earth’s surface and the fourth dimension as the time at which a point was captured [15]. Based on this, a space-filling curve method was developed to index the DGGS grids on an ellipsoidal Earth model, sample tests were run, and queried points were visualized in a Web browser [15]. It shows that DGGS’s structure is advisable to realize a global, integrated point cloud. Follow-up studies may include exploring alternative index mechanisms, multi-dimensional analysis, applicability, dynamic visualization, etc.
Citation
- Purss, M., et al., Datacubes: a discrete global grid systems perspective. Cartographica The International Journal for Geographic Information and Geovisualization, 2019. 54(1): p. 63-71.
- Goodchild, M.F., Reimagining the history of GIS. Annals of GIS, 2018. 24(1): p. 1-8.
- SEDNA. Safe maritime operations under extreme conditions: the Arctic case. 2020 [cited 2020 Jan. 15]; Available from: https://www.sedna-project.eu/.
- EO4wildlife. EO4wildlife project. 2020 [cited 2020 Jan.15]; Available from: http://www.eo4wildlife.eu/.
- Furtado, P. and P. Baumann, Storage of multidimensional arrays based on arbitrary tiling, in Proceedings 15th International Conference on Data Engineering (Cat. No.99CB36337). 1999. p. 480-489.
- Salehi, M., et al. From transactional spatial databases integrity constraints to spatial data cubes integrity constraints. in Proceedings of the 5th International Symposium on Spatial Data Quality. 2007.
- Baumann, P., et al., Datacubes: towards space/time analysis-ready data, in Service oriented mapping - changing paradigm in map production and geoinformation management, J. Doellner, M. Jobst, and P. Schmitz, Editors. 2018, Springer: Cham. p. 269-299.
- Yao, X., et al., Enabling the big earth observation data via cloud computing and DGGS: opportunities and challenges. Remote Sensing, 2019. 12(1).
- Mohamed-Ghouse, Z.S., C. Desha, and L. Perez-Mora, Digital earth in Australia, in Manual of digital earth, H. Guo, M. Goodchild, and A. Annoni, Editors. 2020, Springer Open. p. 683-711.
- Mahdavi-Amiri, A., T. Alderson, and F. Samavati, A survey of digital earth. Computers and Graphics, 2015. 53: p. 95-117.
- Tong, X., et al., An efficient integer coding index algorithm for multi-scale time information management. Data & Knowledge Engineering, 2019. 119: p. 123-138.
- Granell, C., et al., Internet of Things, in Manual of Digital Earth. 2020. p. 387-423.
- ITU, A guide to the Internet of Things infographic. 2018. 2020(Feb. 10).
- Purss, M.B., et al. Applying discrete global grid systems to sensor networks and the Internet of Things. in 2017 IEEE International Geoscience and Remote Sensing Symposium (IGARSS). 2017. IEEE.
- Sirdeshmukh, N., et al., Utilizing a Discrete Global Grid System for handling point clouds with varying locations, times, and levels of detail. Cartographica: The International Journal for Geographic Information and Geovisualization, 2019. 54(1): p. 4-15.